The Comprehensive Guide to Data Labelling Services
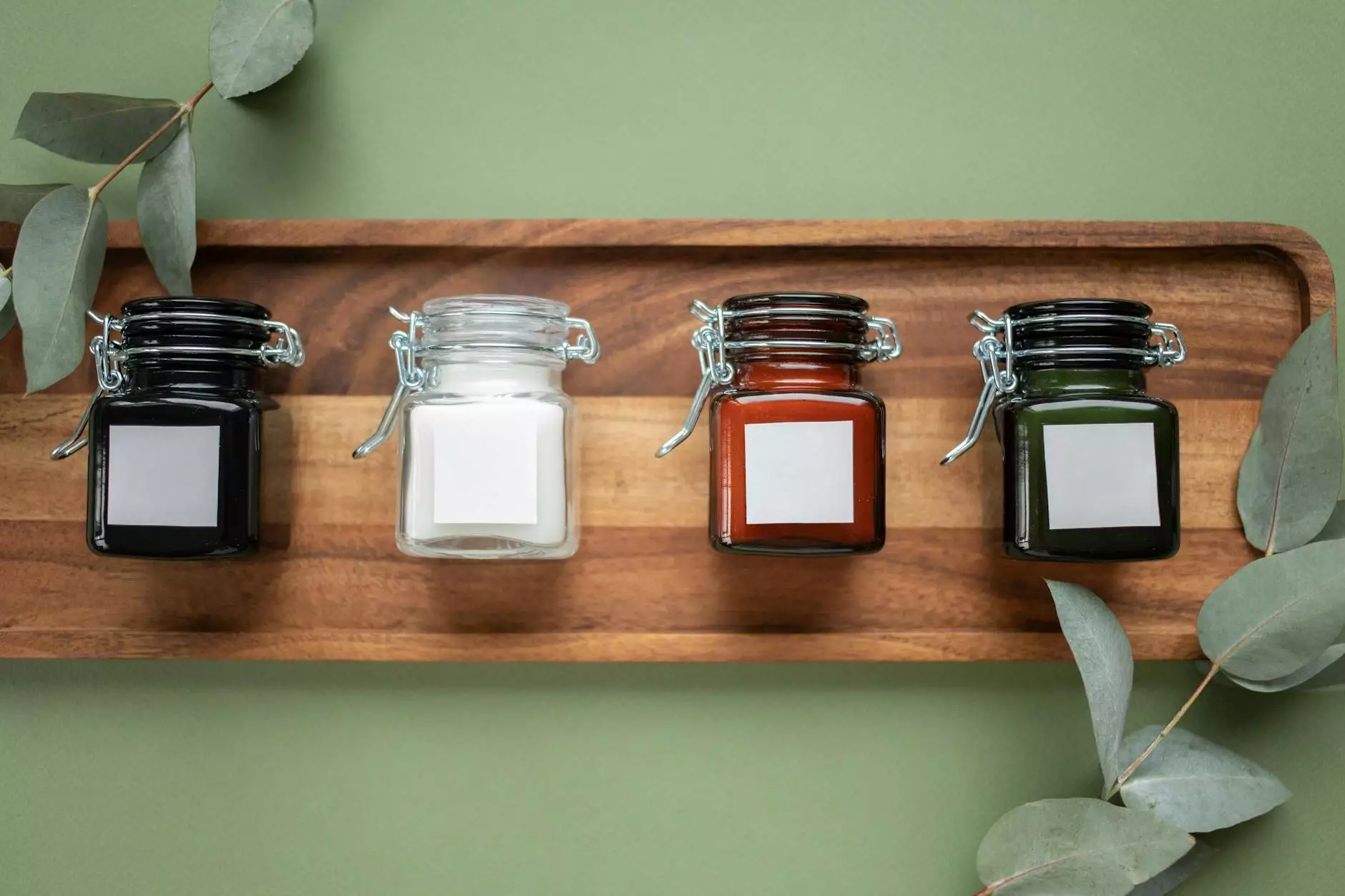
In today’s rapidly evolving technological landscape, data labelling services have emerged as a crucial component for organizations harnessing the power of Artificial Intelligence (AI) and Machine Learning (ML). As businesses increasingly rely on data-driven decision-making, the need for accurate and efficient data labelling is paramount. In this article, we will explore the various facets of data labelling services, their impact on AI and ML projects, and how a platform like Keylabs.ai can assist enterprises in streamlining their data annotation processes.
Understanding Data Labelling Services
At its core, the term data labelling service refers to the process of annotating or tagging data, making it understandable for machine learning models. This service is vital for training algorithms to recognize patterns and make predictions. Without accurately labelled data, an AI model's capability is severely compromised, leading to suboptimal outcomes. Here’s why data labelling matters:
1. The Backbone of AI and ML
Data labelling is fundamental in teaching machine learning algorithms to interpret data. Every image, text, or audio file fed into a model needs proper context, and data labelling provides that context. Annotated datasets serve as the training ground for these algorithms, allowing them to learn and improve over time.
2. Ensuring Accuracy and Efficiency
Quality data labelling services ensure high accuracy in annotations, which is critical for the performance of AI systems. Clean, well-organised datasets lead to efficient model training, reducing the need for re-training and correction which can be time-consuming and costly.
The Process of Data Labelling
Data labelling involves several steps to ensure precision and quality. Here’s an overview of the general workflow:
1. Data Collection
The first step involves gathering raw data, which can range from images and videos to text documents and audio recordings.
2. Data Preparation
This phase includes cleaning and organizing the data, ensuring that it's in a state suitable for annotation. This may involve filtering out irrelevant information or formatting the data correctly.
3. Annotation
Here’s where the actual labelling happens. Annotators label the data according to predefined parameters, creating a structured dataset for machine learning applications.
4. Quality Check
After labelling, it is crucial to perform quality checks. This ensures the accuracy of the annotations and adherence to the project specifications.
5. Final Delivery
Once the data has been labelled and checked for quality, it is delivered back to the client, ready for use in developing and training AI models.
The Importance of Quality in Data Labelling Services
As the demand for data labelling services grows, so does the need for high-quality annotations. Poor annotations can lead to disastrous results in applications such as autonomous vehicles, healthcare diagnostics, and financial forecasting. High-quality data labelling enhances the learning process of AI models, leading to:
- Improved Performance: Well-labelled data allows for more accurate and reliable predictions.
- Reduced Errors: Minimizing inaccuracies prevents potential failures in critical applications.
- Faster Deployment: High-quality labelled data accelerates the training process, enabling quicker implementation of AI solutions.
Types of Data Labelling Services
There are various types of data labelling services suited for different needs. Here are a few common types:
1. Image Labelling
Image labelling involves annotating images for various applications, including object detection, image segmentation, and facial recognition.
2. Video Labelling
This service focuses on annotating video footage for activities, motion tracking, and event recognition, crucial for industries like security and entertainment.
3. Text Annotation
Text annotation encompasses tasks like entity recognition, sentiment analysis, and text classification, vital for natural language processing (NLP) applications.
4. Audio Labelling
Audio labelling identifies features in audio recordings, essential for voice recognition and speech analytics.
Keylabs.ai: Leading the Way in Data Labelling
With a dedicated focus on delivering top-notch data labelling services, Keylabs.ai has positioned itself as a leader in the field. Utilizing state-of-the-art technology and a team of highly skilled annotators, Keylabs.ai provides a robust data annotation platform that streamlines the labelling process and ensures high-quality outputs.
Advantages of Keylabs.ai
- Scalability: Keylabs.ai can handle large volumes of data efficiently, making it ideal for enterprises with extensive datasets.
- Flexibility: The platform supports various data types, from images and text to audio and video.
- Quality Assurance: Every labelled dataset undergoes rigorous quality checks, guaranteeing accuracy and reliability.
- Custom Solutions: Keylabs.ai works closely with clients to provide tailor-made solutions that fit specific project requirements.
Best Practices for Choosing a Data Labelling Service
When selecting a data labelling service, consider the following best practices:
1. Evaluate Expertise and Experience
Choose a provider with a proven track record in the field. Look for case studies or testimonials that demonstrate successful projects and client satisfaction.
2. Inspect Quality Assurance Measures
Ensure the service provider has robust quality assurance practices in place to maintain high standards in data annotation.
3. Consider Turnaround Time
Project timelines are crucial in fast-paced business environments. Opt for a service that can meet your deadlines without compromising on quality.
4. Understand Pricing Structures
Transparent pricing helps prevent unexpected costs. Ensure you understand how pricing works and what factors influence costs.
5. Look for Post-Delivery Support
Post-delivery support is essential for addressing any issues that may arise after receiving the labelled data. Choose a service provider that is responsive and helpful.
The Future of Data Labelling Services
The landscape of data labelling services is transforming with advancements in technology, including artificial intelligence and automation. Here are some trends to watch for:
1. Increased Use of AI in Labelling
The integration of AI in the labelling process promises enhanced efficiency and scalability. While human intervention remains essential for quality, AI can assist by automating repetitive tasks and improving speed.
2. Enhanced Collaboration Tools
Improved collaboration tools will enable teams to work more effectively, sharing insights and feedback quickly, thereby boosting the efficiency of the labelling process.
3. Growth of Distributed Workforces
The trend towards remote work opens up a global talent pool for data labelling. Companies can leverage expertise from diverse locations, leading to improved service delivery.
4. Focus on Ethical Labelling Practices
As data privacy regulations become stringent, ethical considerations in data labelling will take precedence. This will include protecting user data and ensuring compliance with laws.
Conclusion
In conclusion, data labelling services are an indispensable part of the modern AI landscape, providing the essential groundwork for training machine learning models. The accuracy of these services directly impacts the effectiveness of AI systems, making it crucial for businesses to invest in high-quality solutions. Keylabs.ai stands out as a premier data annotation platform, committed to delivering exceptional labelling services that meet diverse business needs. By understanding the importance of data labelling and choosing the right service provider, organizations can unlock the full potential of their data, driving innovation and growth in the increasingly competitive market.